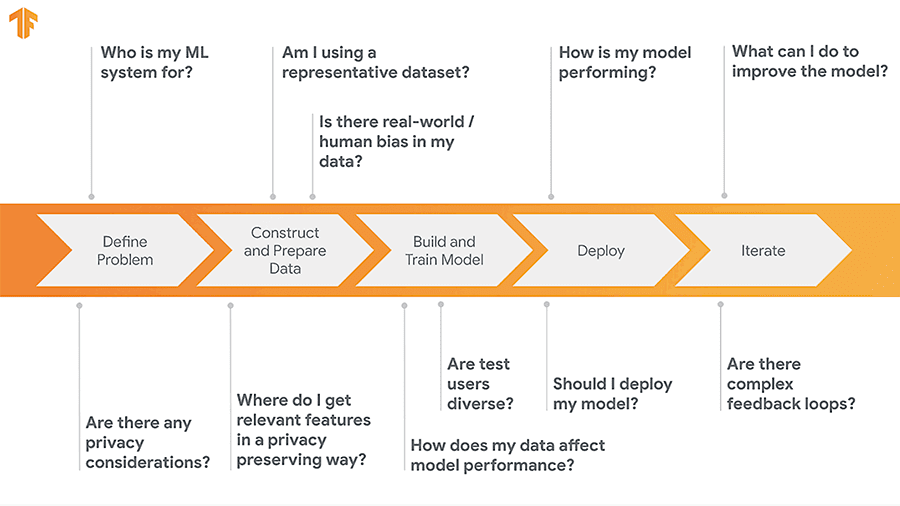
This article will discuss ML, clustering and metadata. It will also discuss the differences between unsupervised and supervised learning. We will also talk about how to use Metadata Registry to store metadata from your ML model. These are important concepts for understanding ML model's. These concepts can be used for better modeling. This article will cover these concepts in greater detail.
ML model metadata
Metadata is a key component of ML models. This allows reproducibility and auditing. A metadata management tool lets you store and retrieve all the data and settings for your model in one location. Metadata can help you identify reused model building steps, assimilate models, and allow for auditing and comparison. ML model metadata includes information such as model type, types of features, preprocessing steps, hyperparameters, metrics, and training/test/validation processes. It also contains information such as the number of iterations, training time, and other details.
This data is stored in a repository. Models can be linked through edge computing devices to this repository. A camera and microphone, for example, can be connected to the ML 400 via Bluetooth communications or a USB cord. The raw input data could be stored in ML model repository 408, and may be associated with labeled tags, expert input, or any other information. You can also store this data in another storage location accessible by the ML engines.
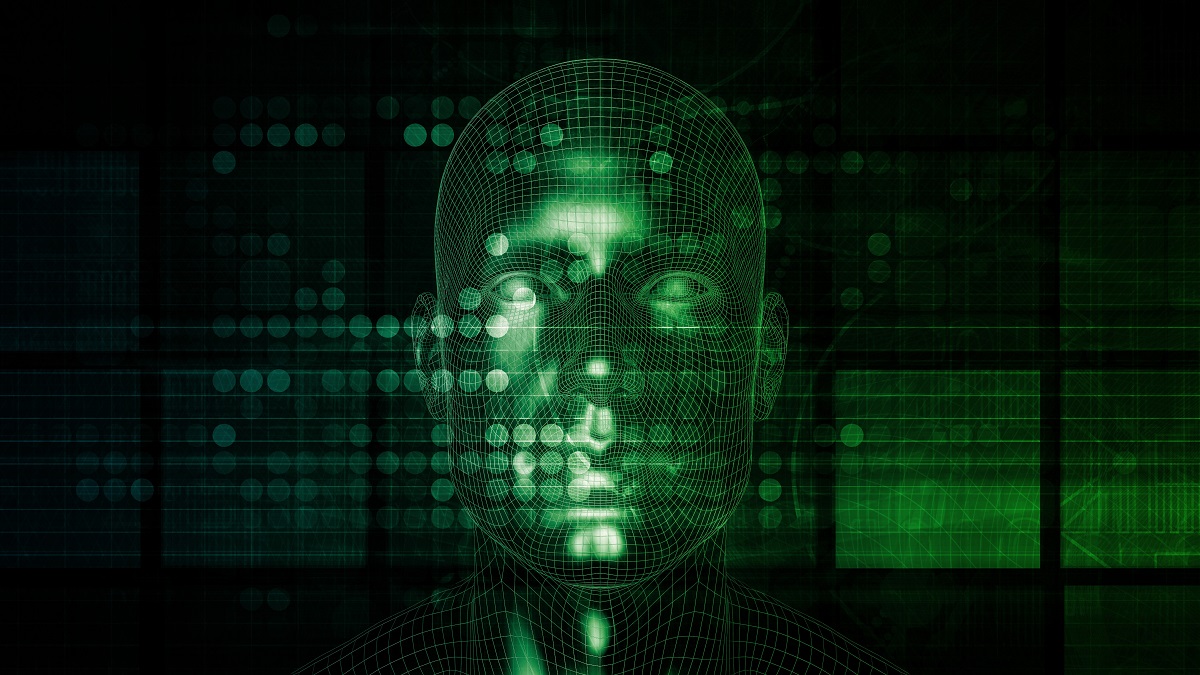
ML model clustering
Clustering ML models is the process of identifying similar examples, and then grouping them together. The data from each feature is combined to create a similarity indicator. One example is a book that has three covers. The algorithm gets more complicated the more features there are. It can identify similar items by analyzing how often the book was purchased. The goal of ML Model Clustering is to identify the most efficient way to group data into groups that will maximize revenues and minimize costs.
When training an ML model, you need to choose an appropriate clustering method. The best way to do this is to train the model on a large dataset. This will make it possible to use the model for predictions about the data you have. Clustering is useful for identifying patterns and structures that exist in data that may otherwise be unrelated. It is especially useful for data science. Predictive analytics requires the use of ML model clustering.
Unsupervised learning vs. supervised
The difference between unsupervised and supervised learning is in how they use data sets with few or none labels. Unsupervised learning is possible without labeling the data. Unlike supervised, which requires humans to add labels to the data. Unsupervised learning can also help with problems such as clustering, anomaly identification, and flagging outliers.
While both algorithms have their merits, supervised learning is more useful in situations where the input data and output data are known. Unsupervised learning allows for greater flexibility and can deal with large volumes of data in real-time. It also helps identify structures in the data, which is critical for many applications, including the segmentation of potential consumers. An unsupervised clustering technique can be used to identify apples that share similar characteristics. This method can be used to tackle complex response variables like stress levels.
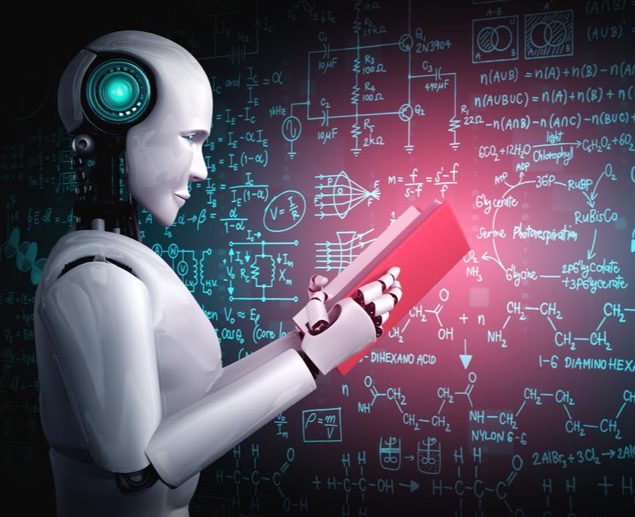
Registry for metadata
The foundation of a semantic Web is a metadata registry. This technology enables Web applications with unambiguous meanings to share information between each other. In order to achieve this, registries will have to be multilingual, both in terms of UI and data. This requirement was incorporated into the design of metadata registry prototypes. There are currently 14 languages that can be supported by the Dublin Core elements set. Initially, six languages were chosen for proof of concept development. These languages were single-byte language sets like Spanish or double-byte sets like Japanese. For proof of concept, however, only a small portion of each prototype could be translated.
A metadata database is a centrally maintained list of terms that are used in an application. A metadata registry stores data that can be linked to schemas used by implementers. The metadata registry also allows computer programs to take advantage of ontologies and utilize them. Reusing existing terms can be done through registries. Using metadata registries is a great way to improve the quality of data that is available for users.
FAQ
Are there any AI-related risks?
Of course. They will always be. AI could pose a serious threat to society in general, according experts. Others believe that AI is beneficial and necessary for improving the quality of life.
The biggest concern about AI is the potential for misuse. Artificial intelligence can become too powerful and lead to dangerous results. This includes things like autonomous weapons and robot overlords.
Another risk is that AI could replace jobs. Many fear that AI will replace humans. Some people believe artificial intelligence could allow workers to be more focused on their jobs.
For instance, economists have predicted that automation could increase productivity as well as reduce unemployment.
How will governments regulate AI?
The government is already trying to regulate AI but it needs to be done better. They must make it clear that citizens can control the way their data is used. Companies shouldn't use AI to obstruct their rights.
They must also ensure that there is no unfair competition between types of businesses. If you are a small business owner and want to use AI to run your business, you should be allowed to do so without being restricted by big companies.
What is the future of AI?
The future of artificial intelligence (AI) lies not in building machines that are smarter than us but rather in creating systems that learn from experience and improve themselves over time.
This means that machines need to learn how to learn.
This would allow for the development of algorithms that can teach one another by example.
You should also think about the possibility of creating your own learning algorithms.
You must ensure they can adapt to any situation.
Is AI the only technology that is capable of competing with it?
Yes, but it is not yet. There have been many technologies developed to solve specific problems. However, none of them can match the speed or accuracy of AI.
How does AI work?
To understand how AI works, you need to know some basic computing principles.
Computers keep information in memory. Computers process data based on code-written programs. The code tells the computer what it should do next.
An algorithm is a set of instructions that tell the computer how to perform a specific task. These algorithms are often written in code.
An algorithm can be thought of as a recipe. A recipe can include ingredients and steps. Each step represents a different instruction. A step might be "add water to a pot" or "heat the pan until boiling."
Statistics
- More than 70 percent of users claim they book trips on their phones, review travel tips, and research local landmarks and restaurants. (builtin.com)
- In the first half of 2017, the company discovered and banned 300,000 terrorist-linked accounts, 95 percent of which were found by non-human, artificially intelligent machines. (builtin.com)
- While all of it is still what seems like a far way off, the future of this technology presents a Catch-22, able to solve the world's problems and likely to power all the A.I. systems on earth, but also incredibly dangerous in the wrong hands. (forbes.com)
- According to the company's website, more than 800 financial firms use AlphaSense, including some Fortune 500 corporations. (builtin.com)
- Additionally, keeping in mind the current crisis, the AI is designed in a manner where it reduces the carbon footprint by 20-40%. (analyticsinsight.net)
External Links
How To
How to set up Google Home
Google Home, an artificial intelligence powered digital assistant, can be used to answer questions and perform other tasks. It uses natural language processing and sophisticated algorithms to answer your questions. Google Assistant can do all of this: set reminders, search the web and create timers.
Google Home can be integrated seamlessly with Android phones. By connecting an iPhone or iPad to a Google Home over WiFi, you can take advantage of features like Apple Pay, Siri Shortcuts, and third-party apps that are optimized for Google Home.
Google Home offers many useful features like every Google product. Google Home will remember what you say and learn your routines. It doesn't need to be told how to change the temperature, turn on lights, or play music when you wake up. Instead, you can simply say "Hey Google" and let it know what you'd like done.
To set up Google Home, follow these steps:
-
Turn on Google Home.
-
Hold the Action button in your Google Home.
-
The Setup Wizard appears.
-
Select Continue
-
Enter your email address.
-
Register Now
-
Google Home is now online